When the pandemic began in early 2020, the first casualty in the retail industry was supply chain. There was chaos, and consumers struggled to find ways to fulfill their daily needs – essential as well as non-essential – in the restricted environment they had access to outside their homes. Gradually, overall disruption in end-to-end supply chain began to show its impact on businesses globally.
On one hand movement of supplies created shortages of certain categories, while on the other, huge inventories were piling up of categories of low priority in the consumer basket. Disruption in logistics of supply chain added to the overall chaos.
Even today, with the end of our pandemic era on the horizon, it’s clear that the overall supply chain planning models have been permanently altered. Over the traumatic period, consumers changed their buying habits and patterns, their baskets got distributed across new shopping channels, and there has been a huge demographic shift in consumer spend.
Also, a large number of new brands emerged, especially in food and beverages. Historic business data pertaining to retail and consumer brands is no longer relevant as a new consumer mix has emerged with new buying habits and patterns.
It’s time to take a fresh look at supply chain strategies and planning models. Brands need to now move away from inventory driven models to a demand-driven approach for inventory planning. That means stock only what your customers are going to buy rather than trying to push inventories onto them.
A demand-driven approach will be the key to not just maintaining optimum inventory load but also to increase full price sales through improved availability. Once inventory is aligned to the demand, it reduces wastage to the lowest, especially of perishable and expiry-date items.
This also creates a big opportunity for food brands to enhance their freshness quotient. This will improve brand value and also enable brands to contribute to environment and sustainability standards.
For the success of the demand-driven model, it is important to access the precise demand of every item at every sales location, for each replenishment cycle. Total demand comprises of factors like smooth demand, intermittent demand and lumpy demand. Each of these factors may have different trends for different categories such as food, daily, fashion, durables etc.
These are further influenced by external demand-drivers such as weather, seasons, festivals, national events, local events, day of the week, day of the month, competition, demographics, micro-economic factors, impact of pandemic and other such unexpected sweeping events or external factors. In any business, the overall demand distribution is generally in a 60:10:10:20 ratio across smooth, lumpy and intermittent influenced by external factors as described above.
In the past, demand-driven models didn’t work because brands were not able to identify demand accurately. Traditionally, most demand planners have been making estimations either on excel sheets or using time-series statistical models to forecast the demand. Most of these estimations were manually tweaked based on human instinct and relied on the experience of the person making them.
The forecasts worked at not more than 60-65% accuracy since there was no scientific way to understand the influence of so many demand-drivers externally while understanding lumpy and intermittent trends at the same time. This is nothing more than identifying smooth demand patterns. In the post-pandemic era, even that has become more complex since historic business data has lost its relevance due to the changes in customer and market behaviour.
This gap of more than 30% in understanding demand is due to the inability to understand the influence of the range of external demand-drivers for each business, especially variances due to lumpy and intermittent demand. To give an example, a brand store in a shopping mall will sell more on a Friday than another store of the same brand on the high street since a mall is likely to have higher footfalls on a big-starrer Friday release in the mall.
Mapping such minor demand variables in every sales location can be critical to measure and understand the real demand. Similarly, a store of the same brand in a corporate park will have different demand patterns and you need to have a different methodology to measure that. Most brands fail to determine sales location-profile based demand and find it difficult to work on demand-driven strategy.
This is where the role of modern technologies come into play! Emerging technologies based on artificial intelligence (AI) and machine-learning (ML) have now built capabilities to create a profile-based library of models to understand the impact of such variables and volatilities of demand.
These models are designed to learn from their own inaccuracies and keep improving their forecasting performance on different parameters. The key is the ability to understand the variations in external conditions, and their impact on the demand in each situation keeps the role of this technology in play.
The AI-ML based engines are great to deliver insights into such complex business requirements. However, if these insights are not put into action at the right time, all the effort and investment will be lost. New use of these AI engines also adds capabilities to read the insights generated and trigger required business actions through a pre-determined process and approval workflow.
This has been proven to deliver a significant impact on aligning performance with business goals. There are case studies where 90-95% accuracy has been achieved in aligning demand with inventory, leading to a direct impact on sales growth, reduction in wastage and improved efficiency in the supply chain.
This article first appeared in The Business Guardian on 25 July 2022.
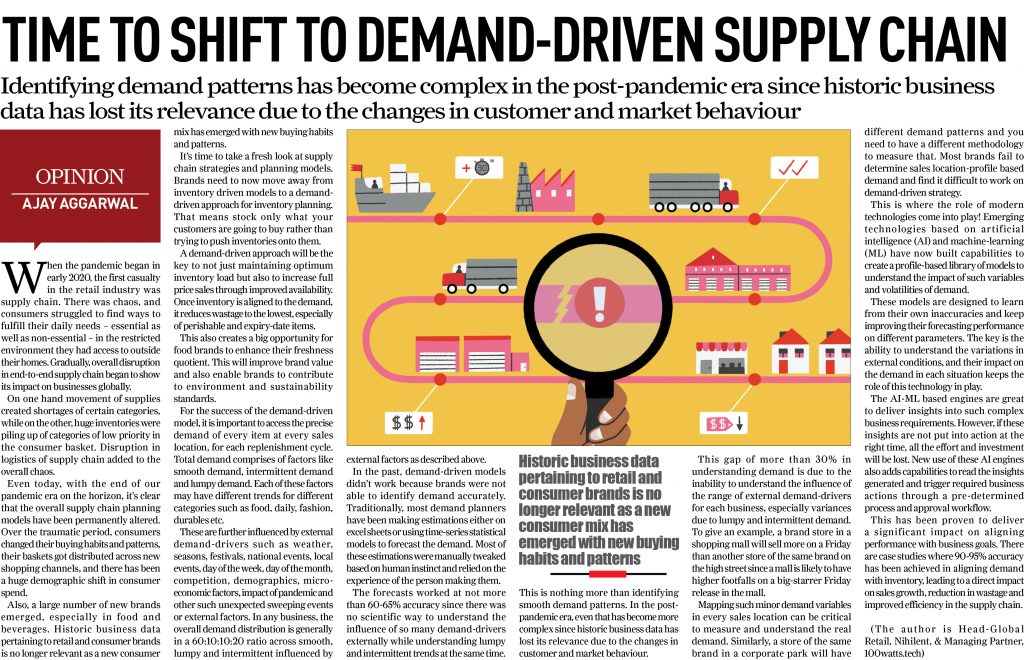